Apple Engineers Show How Flimsy AI ‘Reasoning’ Can Be
For a while now, companies like OpenAI and Google have been touting advanced "reasoning" capabilities as the next big step in their latest artificial intelligence models. Now, though, a new study from six Apple engineers shows that the mathematical "reasoning" displayed by advanced large language models can be extremely brittle and unreliable in the face of seemingly trivial changes to common benchmark problems. Mix It Up In "GSM-Symbolic: Understanding the Limitations of Mathematical Reasoning in Large Language Models"—currently available as a preprint paper—the six Apple researchers start with GSM8K's standardized set of more than 8,000 grade-school level mathematical word problems, which is often used as a benchmark for modern LLMs' complex reasoning capabilities. The fact that such small changes lead to such variable results suggests to the researchers that these models are not doing any "formal" reasoning but are instead “attempt to perform a kind of in-distribution pattern-matching, aligning given questions and solution steps with similar ones seen in the training data.” Don’t Get Distracted Still, the overall variance shown for the GSM-Symbolic tests was often relatively small in the grand scheme of things. Adding in these red herrings led to what the researchers termed "catastrophic performance drops" in accuracy compared to GSM8K, ranging from 17.5 percent to a whopping 65.7 percent, depending on the model tested.
Discover Related
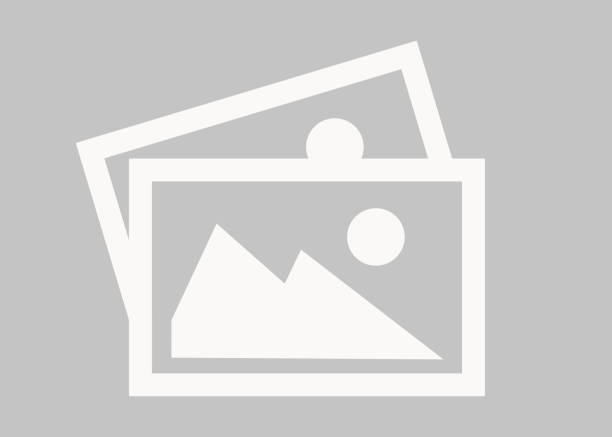
Databricks Has a Trick That Lets AI Models Improve Themselves
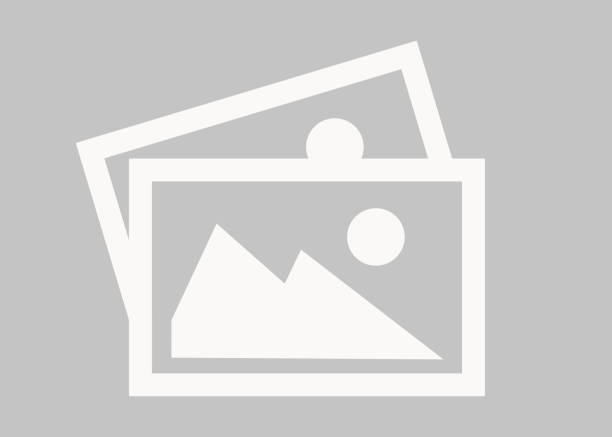
OpenAI Launches GPT-4.5 for ChatGPT—It’s Huge and Compute-Intensive
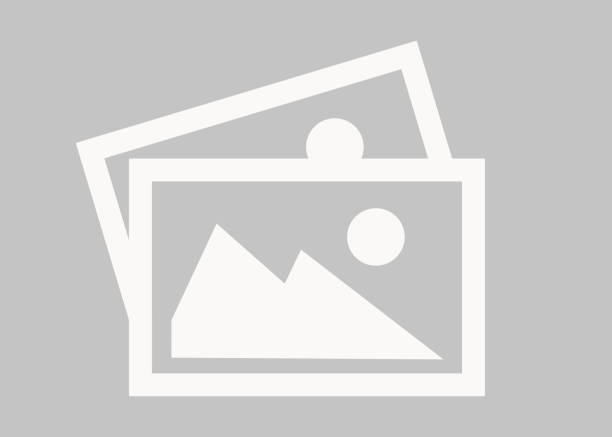
OpenAI rival Anthropic releases its ‘most intelligent’ AI model to date
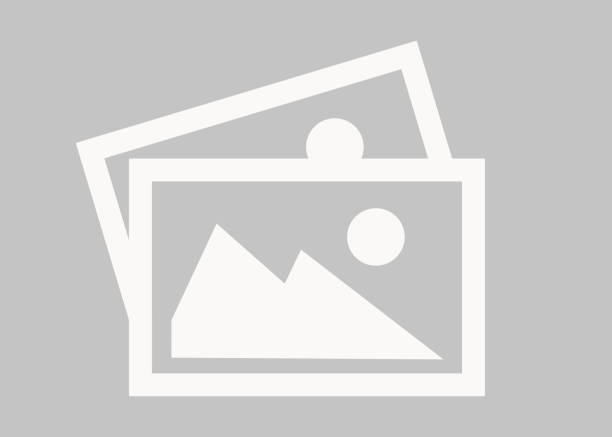
Anthropic Launches the World’s First ‘Hybrid Reasoning’ AI Model
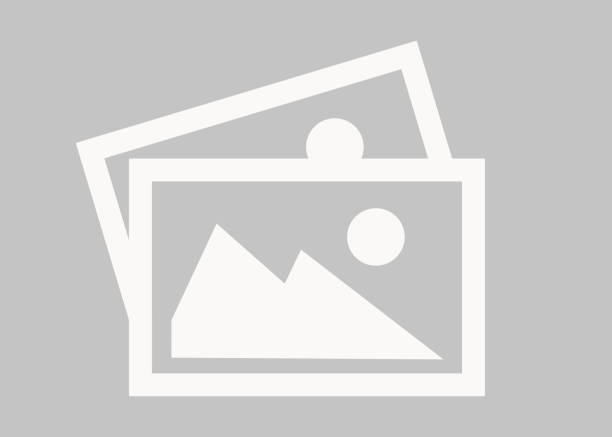
Why AI spending isn't slowing down
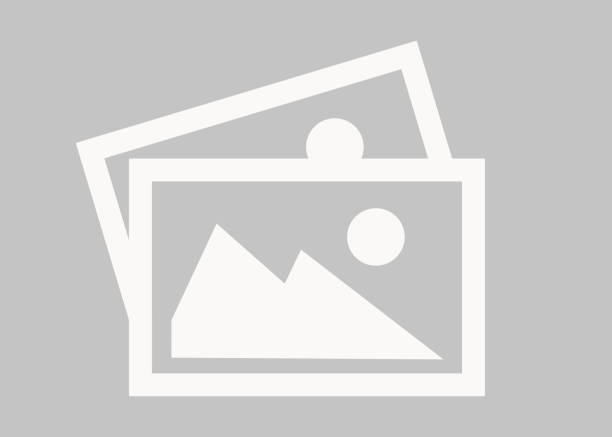
Elon Musk's xAI unveils Grok 3: Users applaud its features and capabilities
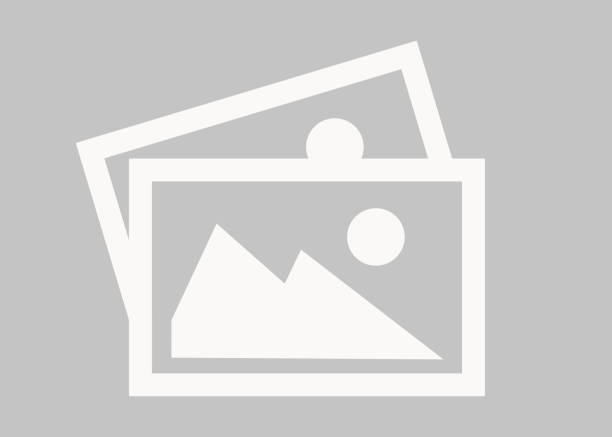
DeepSeek’s R1 may be the first of many AI super-apps to come
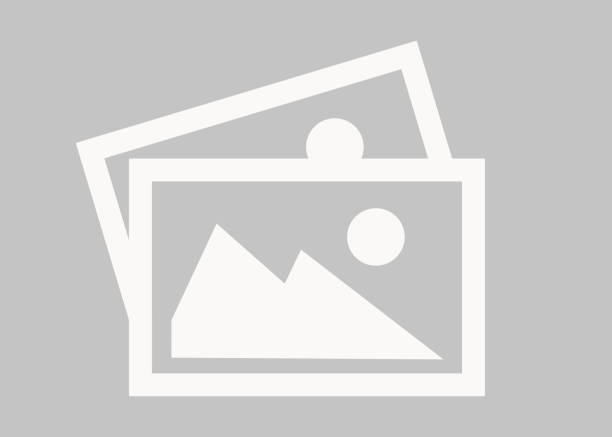
OpenAI releases “newest, most cost-efficient” o3-mini AI model
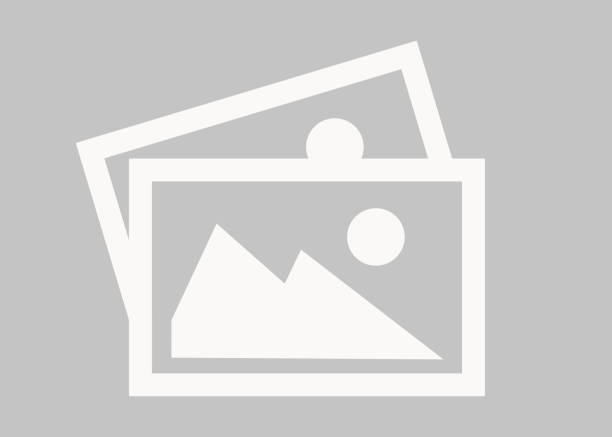
DeepSeek effect? OpenAI rolls out o3-Mini, it’s first reasoning model for free users
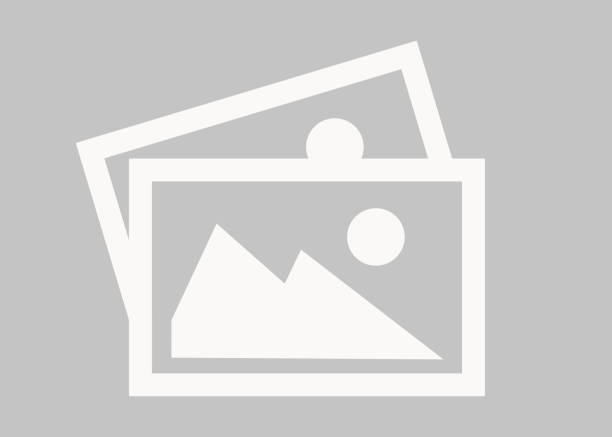
Tim Cook lauds China’s DeepSeek, calls its AI models ‘a good thing’
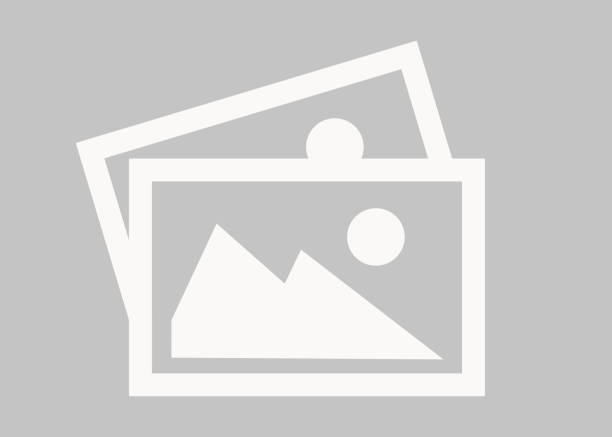
Can DeepSeek disrupt hegemony of US tech giants?
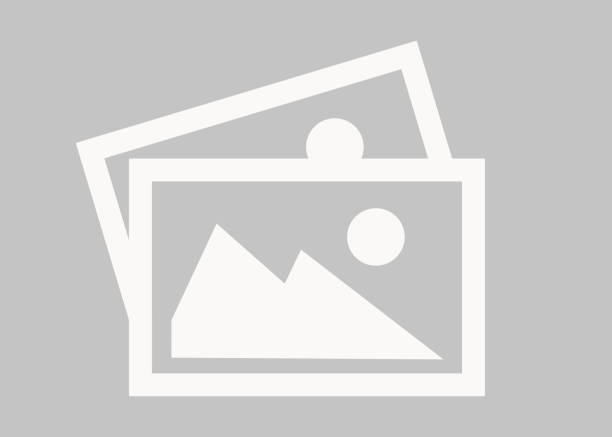
DeepSeek: how a small Chinese AI company is shaking up US tech heavyweights
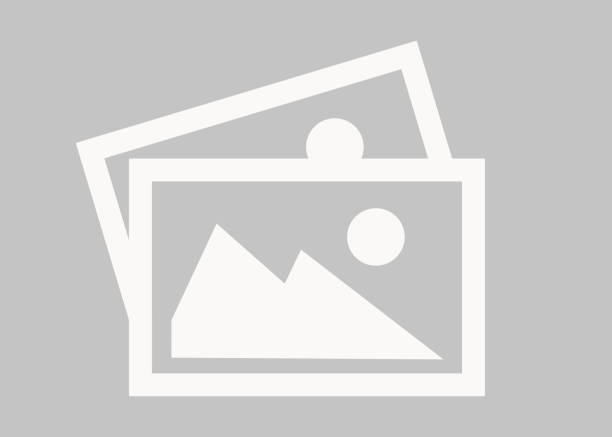
DeepSeek AI: How to use? Why is US alarmed by the rise of China’s AI? Explained
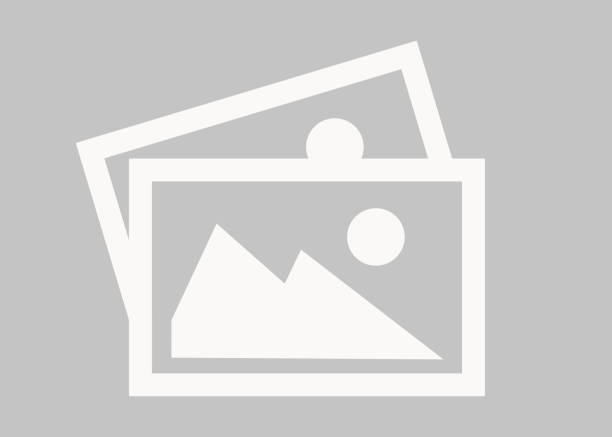
DeepSeek R1's capabilities: How does it differ from ChatGPT and Gemini?
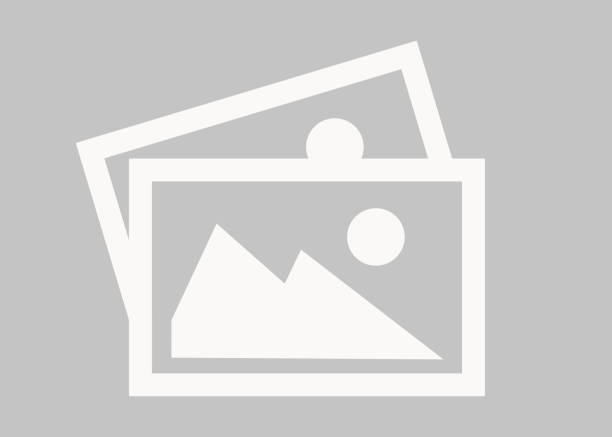
OpenAI’s latest model will change the economics of software
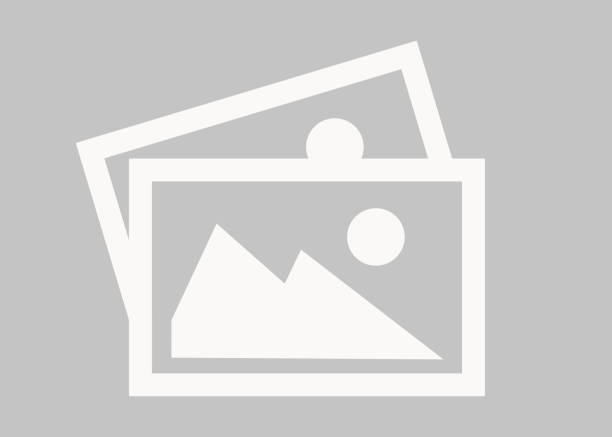
2025 periscope: AI PCs, crypto, 6G, avant-garde tech and what the industry expects
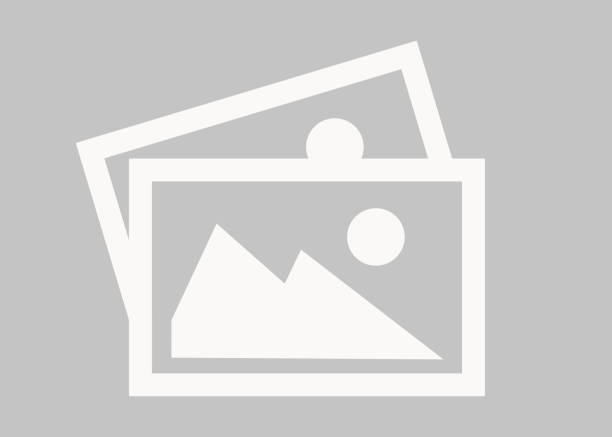
From Gemini, Claude to Llama: How AI titans shaped the industry in 2024
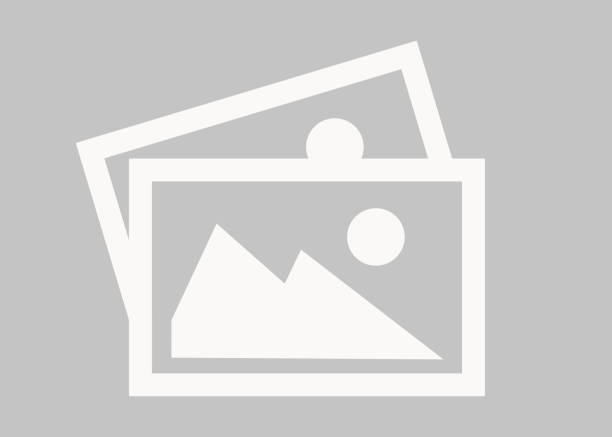
Google Gemini 2.0 Flash Thinking AI Model Unveiled With Advanced Reasoning Abilities
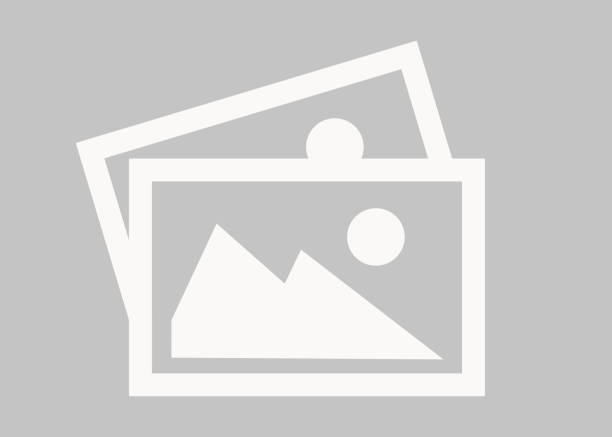
The next great leap in AI is behind schedule and crazy expensive
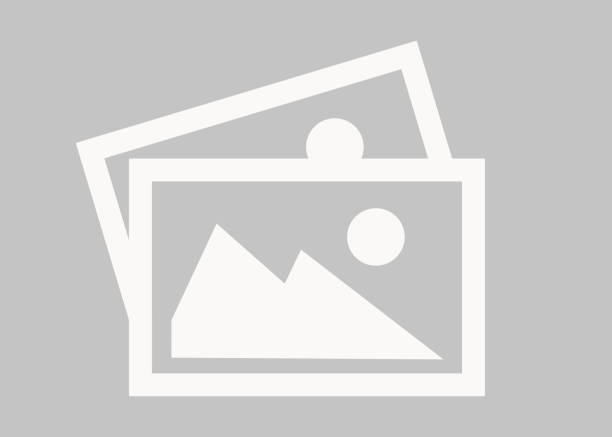
Microsoft introduces Phi-4 AI model, surpassing Gemini Pro 1.5 in mathematical reasoning
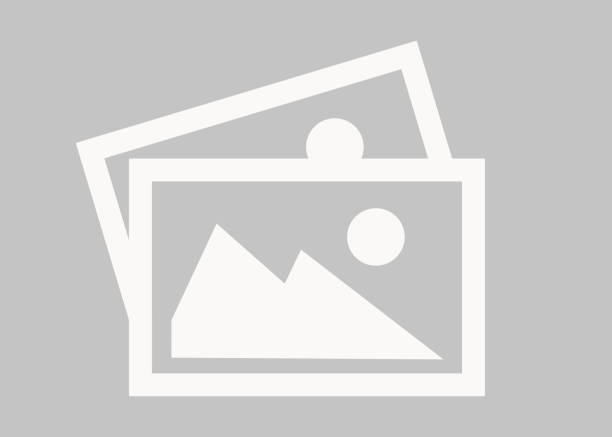
Google reveals the ‘next generation’ of its Gemini AI and promises huge advancements
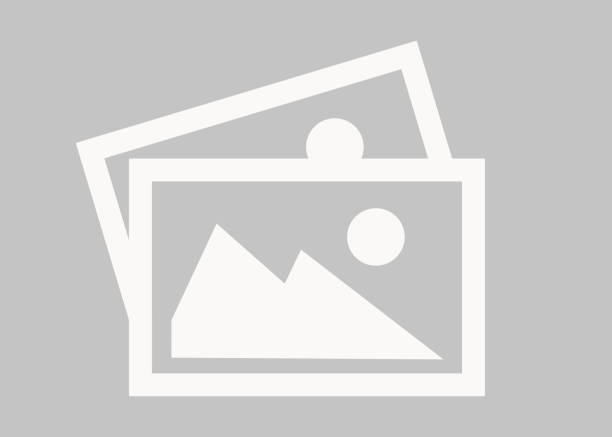
Google reveals the ‘next generation’ of its Gemini AI and promises huge advancements
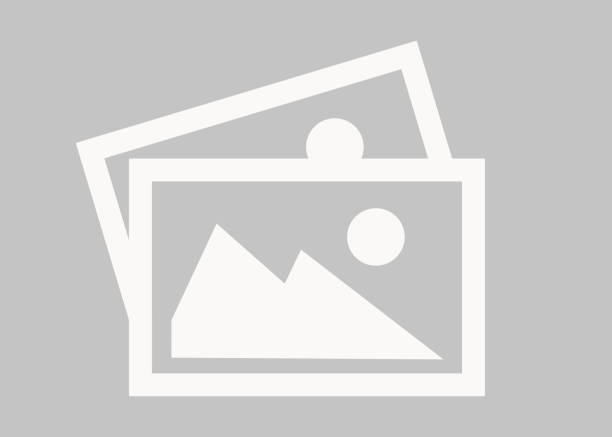
Indian software majors need a clear strategy to ride the AI wave
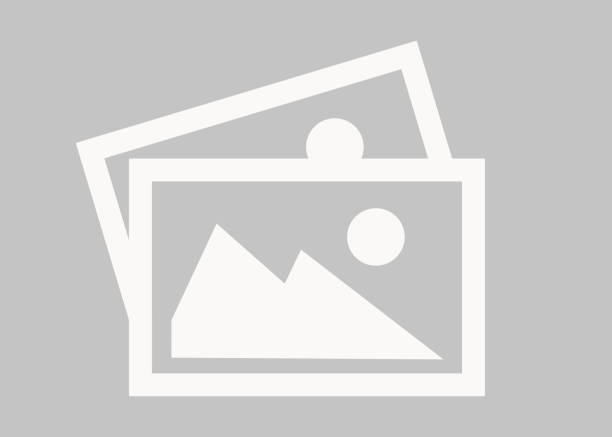
OpenAI and rivals look for new ways to train AI as current methods show limitations
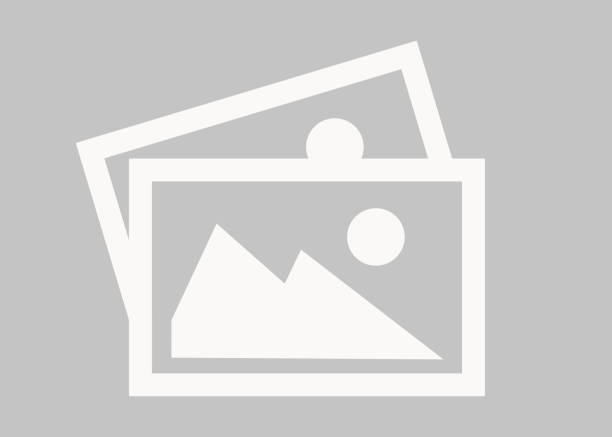